Symposiums--SIGSAC China(Venue : Wanda Realm )
The ACM TURC 2023 (SIGSAC CHINA) conference is leading international forum for academia, government, and industry to present novel research results in various practical and theoretical aspects of cybersecurity. All topic areas related to cyber security and data privacy are within the scope. The ACM TURC 2023 (SIGSAC CHINA) is technically supported by ACM SIGSAC China, which is committed to the research in the fields of security, audit and control, and is the China branch of the world's largest computer security research organization.
Organizers
Forum Chairs:
Qian Wang (Wuhan University)
Deqing Zou (Huazhong University of Science and Technology)
TPC Chairs:
Qi Li(Tsinghua University, China)
Haojin Zhu (Shanghai Jiaotong University)
Zhen Ling (Southeast University)
Zhe Liu (Nanjing University of Aeronautics and Astronautics)
Local Chair:
Shengshan Hu (Huazhong University of Science and Technology))
Ming Wen (Huazhong University of Science and Technology)
Meeting schedule
Date(2023-07-29)Location: Multifunction Hall 4 | ||||
Time | Session | Title | Speaker | Host |
13:30-13:40 | Opening | Welcome Speech | Kui Ren | Deqing Zou |
13:40-14:35 | Keynote | AI and DevSecOps | Yang Liu (Nanyang Technological University) | |
14:35-14:45 | Break | |||
14:45-15:05 | Invited Talk | MagBackdoor: Beware of Your Loudspeaker as A Backdoor For Magnetic Injection Attacks | Feng Lin(Zhejiang University) | Zhen Ling, Ming Wen |
15:05-15:25 | Invited Talk | Proxy re-encryption for data sharing in cloud computing | Chunpeng Ge(Shandong University) | |
15:25-15:45 | Invited Talk | Introduction to the Academic Research on Internet Domain Name System Security | Baojun Liu(Tsinghua University) | |
15:45-15:55 | Break | |||
15:55-16:15 | Invited Talk | Risk-Aware Based Safe Reinforcement Learning | Xiaozhen Lu(Nanjing University of Aeronautics and Astronautics) | |
16:15-16:35 | Invited Talk | Automatic cryptanalysis and design of symmetric-key primitives | Ling Sun(Shandong University) | |
16:35-16:55 | Invited Talk | Trustworthy Large Language Model | Bingzhe Wu (Tencent AI Lab) |
Date(2023-07-30)Location: Multifunction Hall 4 | ||||
Time | Session | Title | Speaker | Host |
13:30-14:25 | Keynote | Can Federated Learning Really Preserve Privacy? | Baochun Li (Toronto University) | Qian Wang |
14:25-14:35 | Break | |||
14:35-14:55 | Invited Talk | Robust Detection of Malicious Encrypted Traffic in Diverse Network Environments | Jiahao Cao(Tsinghua University) | Guoxing Chen,Shengshan Hu |
14:55-15:15 | Invited Talk | Recent Progress in Private Information Retrieval | Mingxun Zhou(CMU | |
15:15-15:25 | Break | |||
15:25-15:45 | Invited Talk | De-annoymization Attacks in Virtual Reality Scenario | Yan Meng(Shanghai Jiaotong University) | |
15:45-16:05 | Invited Talk | Exploring the Security Implications of Cross-Layer Interactions in Network Protocols | Xuewei Feng(Tsinghua University) | |
16:05-16:25 | Invited Talk | Analyzing Ground-Truth Data of Mobile Gambling Scams | Geng Hong(Fudan University) |
Keynote Speaker
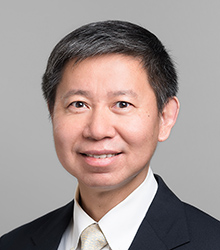
Keynote: Can Federated Learning Really Preserve Privacy?

Baochun Li
Professor at the University of Toronto, IEEE Fellow
Abstract:As an emerging distributed machine learning paradigm, federated learning allows clients to train machine learning models collaboratively with private data, without transmitting them to the server. Though federated learning is celebrated as a privacy-preserving paradigm of training machine learning models, recent work in the literature suggested that sharing gradients with the server may lead to the potential reconstruction of raw private data, such as images and texts, used in the training process. The discovery of this new attack, known as Deep Leakage from Gradients (DLG), has stimulated a recent line of research to improve the attack efficiency and to provide stronger defenses against these known DLG-family attacks. However, before designing for even more efficient and effective defense mechanisms, we begin to have second thoughts on how severe the threat is in practice, even without any defense mechanisms in place. Existing works focused on reconstructing raw data from known gradients or model weights in ideal settings, rather than considering practical settings in federated learning. In this talk, I will introduce some of our recent discoveries that the effectiveness and efficiency of existing gradient leakage attacks are weakened by a substantial margin in standard federated learning settings, where clients send model updates rather than gradients, perform multiple local training iterations over local data with a non-i.i.d. distribution, and initialize model weights normally. I will also present our new light-weight defense mechanism, called Outpost, that provides sufficient and self-adaptive protection throughout the federated learning process against time-varying levels of privacy leakage risks. This is a joint work with Fei Wang, who is currently a Ph.D. student at the University of Toronto.
Bio:Baochun Li received his B.Engr. degree from the Department of Computer Science and Technology, Tsinghua University, China, in 1995 and his M.S. and Ph.D. degrees from the Department of Computer Science, University of Illinois at Urbana-Champaign, Urbana, in 1997 and 2000. Since 2000, he has been with the Department of Electrical and Computer Engineering at the University of Toronto, where he is currently a professor. He holds the Bell Canada Endowed Chair in Computer Engineering since August 2005. His current research interests include cloud computing, security and privacy, distributed machine learning, federated learning, and networking. Dr. Li has co-authored more than 450 research papers, with a total of over 24000 citations and an H-index of 87, according to Google Scholar Citations. He was the recipient of the IEEE Communications Society Leonard G. Abraham Award in the Field of Communications Systems in 2000, the Multimedia Communications Best Paper Award from the IEEE Communications Society in 2009, the University of Toronto McLean Award in 2009, and the Best Paper Award from IEEE INFOCOM in 2023. He is a Fellow of the Canadian Academy of Engineering and a Fellow of IEEE.
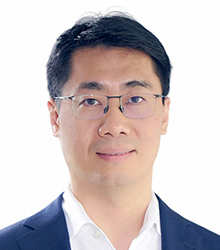
Keynote: AI and DevSecOps

Yang Liu
Professor and University Leadership Forum Chair at the School of Computer Science and
Engineering
Nanyang Technological University
Abstract:DevSecOps entails the systematic integration of security testing throughout all phases of the software development process. The objective is to automate the security expertise of human professionals by employing tools, thereby enabling early identification and resolution of security concerns during the early phase of the development life cycle. However, the effectiveness of DevSecOps greatly relies on the capabilities of intelligent tools to simulate or potentially replace security experts. With the emergence of Artificial Intelligence and Generative Computing (AIGC), a new means to accomplish this objective is now available. In this presentation, I will discuss recent endeavors in utilizing AI within the realm of DevSecOps, specifically in the domains of Static Application Security Testing (SAST), Dynamic Application Security Testing (DAST), and Penetration Testing. Moreover, I will outline potential avenues for employing AIGC across diverse applications, including the construction of specialized large language models tailored to specific domains.
Bio:Dr. Liu Yang is currently a full professor in Nanyang Technological University, director of the cybersecurity lab, Program Director of HP-NTU Corporate Lab and Director of the National Satellite of Excellence of Singapore and Trustworthy AI Center of Singapore. In 2019, he received the University Leadership Forum Chair professorship at NTU. Dr. Liu specializes in software engineering, cybersecurity and artificial intelligence. His research has bridged the gap between the theory and practical usage of program analysis, data analysis and AI to evaluate the design and implementation of software for high assurance and security. Many of his research has been successfully commercialized. By now, he has more than 450 publications in top tier conferences and journals, and 20 best paper awards and one most influence system award in top software engineering conferences. He has received a number of prestigious awards including MSRA Fellowship, TRF Fellowship, Nanyang Assistant Professor, Tan Chin Tuan Fellowship, Nanyang Research Award, ACM Distinguished Speaker, NRF Investigatorship and NTU Innovator (Entrepreneurship) Award.
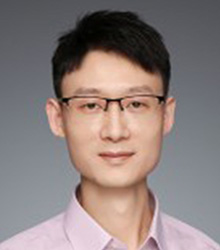
Topic:MagBackdoor: Beware of Your Loudspeaker as A Backdoor For Magnetic Injection Attacks

Feng Lin
A Professor with the School of Cyber Science and Technology, College of Computer Science and Technology, Zhejiang University, China.
Abstract:Smart voice has become a key connection between humans and machines for smart homes, voice assistants, and intelligent industries, due to its hand-free human-computer interaction characteristic. However, these voice interface products give rise to new risks. This paper unveils the backdoor vulnerability of the loudspeaker within internal audio systems, which can be exploited through magnetic injection attacks. Our research is the first to uncover the vocalization mechanism of loudspeakers when subjected to dynamic magnetic fields. Specifically, the modulated magnetic field can compel the speaker to emit malicious commands into the voice interaction system, causing user privacy leakage and property damage. We exploit the knowledge of integrated hardware design to elaborate an attacking prototype that can be discreetly embedded within a standard charging socket. This device emits highly-penetrating magnetic fields, thereby infiltrating and activating malicious tasks on nearby smart voice devices. The magnetic attack poses a new threat to electronic devices, not only expanding attack dimensions but also bringing new inspiration to hardware security design.
Bio:His current research interests include autonomous driving security, Internet of Things security, AI security and Biometrics. He has published more than 100 research papers in mobile computing and security areas, including S&P, CCS, NDSS, MobiCom, MobiSys, SenSys, UbiComp, Infocom. Dr. Lin was a recipient of the ACM SIGSAC China Rising Star Award, the Best Paper Awards from ACM MobiSys'20, IEEE Globecom'19, IEEE/ACM CHASE'22, IEEE BHI'17, the Best Demo Award from ACM HotMobile'18, and the Best Paper Award Nomination from ACM SenSys'21 and IEEE Infocom'21. He serves as an editor for IEEE Network, TPC Chair of ACM Morse'22, and TPC member of MobiCom, SenSys, MobiHoc, ICDCS, etc.
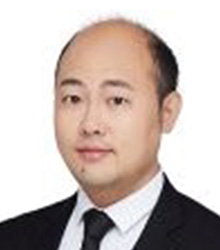
Topic:Proxy re-encryption for data sharing in cloud computing

Chunpeng Ge
A Professor of the School of Software, Shandong University.
Abstract:Cloud computing has becoming the dominant information infrastructure since it eliminates expensive information system construction and local data maintenance. Data security and privacy are important issues in cloud computing. This report focuses on the cloud data security sharing technology based on proxy re-encryption technology, introduces the development of proxy re-encryption technology in detail from the basic concepts, properties, security and other aspects of proxy re-encryption technology, and introduces some latest research results of proxy re-encryption technology . Finally, the report discusses potential future directions and trends for cloud data security and privacy.
Bio:His research interests include data security and privacy protection in cloud computing, and blockchain. He has published more than 60 papers in prestigious journals and conferences such as WWW, IEEE TDSC, TPDS, and DCC. He is an editorial board member of the journal Computer Standards & Interfaces and a member of the program committee of more than 30 international conferences. He has undertooken many NSFC, ministry of science and technology, key research and development projects. The relevant research results have been applied in many military industries, enterprises and other fields. He was award best paper awards of many international academic conferences such as ACISP2022 and NSS 2019.
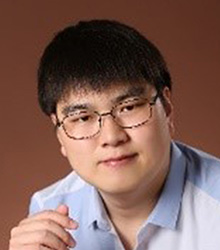
Topic:Introduction to the Academic Research on Internet Domain Name System Security

Baojun Liu
An Associate Professor and PhD supervisor at the Institute of Network Sciences and Cyberspace, Tsinghua University.
Abstract:As one of the key information infrastructures of the Internet, the vast majority of upper-layer network services rely on the domain name system to locate resources. Based on a series of cutting-edge academic achievements in the international network security field in recent years, this report sorts out the dynamic development of academic research on domain name system security, introduces some design and implementation flaws of domain name protocols with important influence, and discusses possible trends in future domain name security research.
Bio:Dr. Liu Baojun's main research areas include: network infrastructure security, Internet measurement and security analysis. He has won important international academic awards such as the Outstanding Paper Award of NDSS Conference, the Best Paper Award of DSN Conference, and the Internet Society Network Research Application Award.
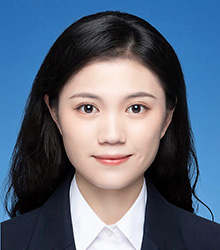
Topic:Risk-Aware Based Safe Reinforcement Learning

Xiaozhen Liu
An Associate Professor with the College of Computer Science and Technology, Nanjing University of Aeronautics and Astronautics, Nanjing, China
Abstract:With the rapid requirements of anti-jamming communications and privacy protection, wireless networks have to design the efficient security mechanisms against malicious attacks such as eavesdropping and jamming. In this report, we will discuss a risk-aware based safe reinforcement learning, which uses hierarchical structure, safe exploration, and safety guide to improve the learning efficiency and security performance and reduce the short-term risk. This algorithm designs a selection priority-based hierarchical structure to compress the action dimensions and formulates the security constraint-based risk avoidance mechanism to avoid risky policies that cause severe network disasters. The algorithm also designs two deep neural networks to evaluate the long-term expected reward and risk values, so as to guide the learning agent to avoid short-term risky policies and further improve the learning speed. As the case study, the effectiveness of the designed algorithm has been verified in the anti-jamming unmanned aerial vehicle system and a cargo transportation system.
Bio:Her research interests include reinforcement learning, network security, and wireless communications. She was a recipient of the ACM SIGSAC China Rising Star Award in 2022, the Best Student Paper Award for ML4CS 2019, and the Excellent Paper Award for CWSN 2020.
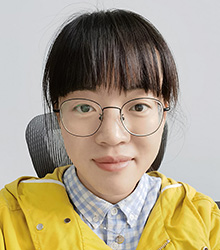
Topic:Automatic cryptanalysis and design of symmetric-key primitives

Ling Sun
Dr. Ling Sun is affiliated with Shandong University.
Abstract:Cryptanalysis of symmetric-key primitives has evolved over the past thirty years, and a variety of methods are now available. At this stage, the security of a cipher can only be demonstrated after it has been evaluated against multiple attack methods. On the one hand, the security analysis of symmetric-key ciphers is a difficult and time-consuming task; on the other hand, ensuring the efficient delivery of trustworthy security evaluation results for novel primitives is becoming an increasingly important factor in cipher design. This presentation will feature the most recent findings in automatic cryptanalysis and the design of symmetric-key primitives.
Bio:Her research interests include cryptanalysis, design of symmetric-key primitives, and machine learning applications in cryptanalysis. She has published over 20 papers in CCF journals/conferences in the field of cryptanalysis and design of ciphers over the past five years, and 14 of those papers are in the top five international cryptographic conferences EUROCRYPT, ASIACRYPT, and FSE. She was awarded the First Prize of National Science and Technology Progress (2020, ranked 12th), the First Prize of National Cryptographic Science and Technology Progress (2017, provincial level, ranked fifth), the Second Prize of Cryptographic Innovation Award of the Chinese Association for Cryptologic Research, the IET Premium Awards 2022, and more.
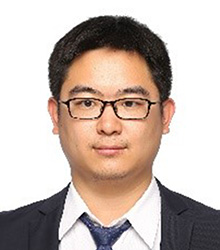
Topic:Robust Detection of Malicious Encrypted Traffic in Diverse Network Environments

Jiahao Cao
A Research Assistant Professor at the Institute of Network Sciences and Cyberspace, Tsinghua University.
Abstract:As the majority of Internet traffic is encrypted by the Transport Layer Security (TLS) protocol, recent advances leverage Deep Learning (DL) models to conduct encrypted traffic classification by automatically extracting complicated and informative features from the packet length sequences of TLS flows. Though existing DL models have reported to achieve excellent classification results on encrypted traffic, we conduct a comprehensive study to show that they all have significant performance degradation in real diverse network environments. After systematically studying the reasons, we discover the packet length sequences of flows may change dramatically due to various TCP mechanisms for reliable transmission in varying network environments. Thereafter, we propose Rosetta to enable robust TLS encrypted traffic classification for existing DL models. It leverages TCP-aware traffic augmentation mechanisms and self-supervised learning to understand implict TCP semantics, and hence extracts robust features of TLS flows. Extensive experiments show that Rosetta can significantly improve the classification performance of existing DL models on TLS traffic in diverse network environments.
Bio:His main research interests include software-defined networking security, network traffic analysis, and routing security. He has published 10 papers in top conferences and journals in the field of networking and security, such as NDSS, CCS, USENIX Security, and TIFS. He has received several awards, including the 2021 ACM SIGSAC China Doctoral Dissertation Award, the 2021 Tsinghua Shuimu Scholar Award, the 2019 USENIX Security Student Travel Grant, and the 2017 EAI SecureComm Best Paper Award.
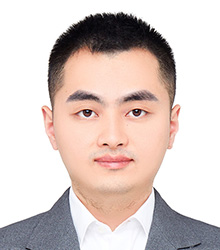
Topic:Trustworthy Large Language Model

Bingzhe Wu
Senior researcher at Tencent AI LAB
Abstract:The rapid development of digital economy has led to the emergence of various black and shadow internet industries, which pose potential risks that can be identified and managed through digital risk management (DRM) that uses different techniques such as machine learning and deep learning. The evolution of DRM architecture has been driven by changes in data forms. However, the development of AI-generated content (AIGC) technology, such as ChatGPT and Stable Diffusion, has given black and shadow industries powerful tools to personalize data and generate realistic images and conversations for fraudulent activities. This poses a challenge for DRM systems to control risks from the source of data generation and to respond quickly to the fast-changing risk environment. This paper aims to provide a technical analysis of the challenges and opportunities of AIGC from upstream, midstream, and downstream paths of black/shadow industries and suggest future directions for improving existing risk control systems. The paper will explore the new black and shadow techniques triggered by generative AI technology and provide insights for building the next-generation DRM system 1.
Bio:His research direction is trustworthy AI, including privacy-preserving machine learning, trust large models, and uncertainty estimation. Graduated from the Department of Mathematics, Peking University with a bachelor's degree, and a Ph.D. from the Department of Computer Science, Peking University. Published more than 30 papers in ICML, ICLR, KDD, NeurlPS, HPCA, ISCA, CVPR and other top conferences. He has won many honorary titles such as ACM China Information Security Society Outstanding Doctorate, China Electronics Education Association Outstanding Doctorate, Apple Doctoral Scholarship (only one in mainland China every year), Beijing Outstanding Graduate and many other honorary titles. Participated in the development of the IEEE P2830 Shared Machine Learning Standard as committee secretary.
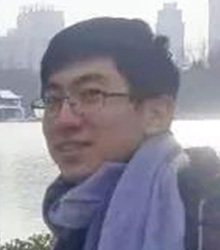
Topic:Recent Progress in Private Information Retrieval

Mingxun Zhou
A PhD student in the Computer Science Department at Carnegie Mellon University, advised by Elaine Shi and Giulia Fanti.
Abstract:Private Information Retrieval(PIR) is a cryptographic primitive that allows a client to access public data without leaking the access history. Researchers have achieved the theoretical optimal results in terms of reducing client computation and communication costs in the classical model. Unfortunately, the per-query server computation cost has been proven to be at least of the order of linearly scanning the whole database in this model. Recently, the Preprocessed PIR model has been proposed and the existence of sub-linear server-cost algorithms has been proved. Our theoretical work (Eurocrypt 2023) constructed a nearly-optimal algorithm in this model. However, without a stronger assumption such as the existence of multiple non-colluding servers, a truly practical sub-linear PIR algorithm is still unknown. Until recently, we proposed the first truly practical single-server sub-linear PIR algorithm, Piano. Piano does not require any heavy-weight cryptographic primitives and is extremely easy to implement. It is sufficiently efficient for practical usage: given a 100GB database, a single query only takes less than 40ms computation time, which is 100x faster than previous single-server PIR schemes. This talk will not assume any background on cryptography. It will be based on the speaker's two recent collaborative works with Andrew Park, Elaine Shi, Wenting Zheng, Yiannis Tselekounis and Wei-Kai Lin.
Bio:His research focuses on privacy-preserving algorithm design, including differential private algorithms and cryptography. He also has research work on Blockchain technology, P2P network.
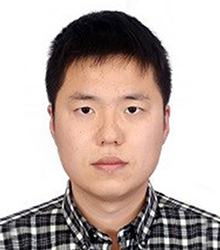
Topic:De-annoymization Attacks in Virtual Reality Scenario

Yan Meng
A Research Assistant Professor in the Department of Computer Science and Engineering at Shanghai Jiao Tong University.
Abstract:Virtual reality (VR) can provide users with an immersive experience in the metaverse. One of the most promising properties of VR is that users’ identities can be protected by changing their physical world appearances into arbitrary virtual avatars. However, recent proposed de-anonymization attacks demonstrate the feasibility of recognizing the user’s identity behind the VR avatar’s masking. In this talk, we propose AvatarHunter, a non-intrusive and user-unconscious de-anonymization attack based on victims’ inherent movement signatures. AvatarHunter imperceptibly collects the victim avatar’s gait information via recording videos from multiple views in the VR scenario without requiring any permission. A Unity-based feature extractor is designed that preserves the avatar’s movement signature while immune to the avatar’s appearance changes. Real-world experiments are conducted in VRChat, one of the most popular VR applications. The experimental results demonstrate that AvatarHunter can achieve attack success rates of 92.1% and 66.9% in closed-world and open-world avatar settings, respectively, which are much better than existing works.
Bio:His research focuses on IoT security, voice interface security, and privacy policy analysis. He has published 13 CCF-A/SCI-Q1 research papers, mainly in CCS, USENIX Security, INFOCOM, TDSC, and TMC. He won the Best Paper Award from the SocialSec in 2015. He is the recipient of the 2022 ACM China Excellent Doctoral Dissertation Award.
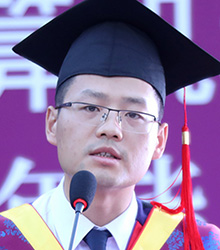
Topic:Exploring the Security Implications of Cross-Layer Interactions in Network Protocols

Xuewei Feng
Dr. Feng is currently employed at the Academy of Military Science, specializing in the field of network security.
Abstract:During the transmission of packets, the TCP/IP protocol stack necessitates dynamic cross-layer interaction among its various protocol layers. Regrettably, a range of security concerns arising from ambiguity and information leakage within this process has not garnered sufficient attention. This report extensively examines and analyzes this problem, meticulously outlining four typical security issues that persist within the TCP/IP protocol stack during cross-layer interaction. Additionally, a comprehensive defense solution is proposed to mitigate these vulnerabilities systematically.
Bio:As the primary author, his research accomplishments have been published in prestigious conferences within the international information security realm. In recognition of his work, he was nominated for the Best Paper Award at CCS'2020. His contributions have garnered recognition and appreciation from various security communities and equipment manufacturers, such as Wi-Fi Alliance, Linux, Github, Qualcomm, Huawei, Alibaba, Ruijie, H3C, and others. Moreover, his findings have been implemented through collaborations with CNCERT, Huawei, etc.
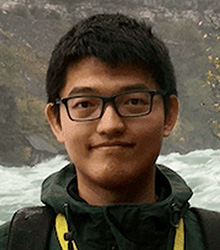
Topic:Analyzing Ground-Truth Data of Mobile Gambling Scams

Geng Hong
An Assistant Proferssor at the Certer for International Governance of Cyberspace, Fudan University.
Abstract:With the rapid development of the mobile Internet, gambling scam are becoming increasingly severe. Scammers set up fake gambling platforms on mobile applications, use instant messaging software to disseminate fraudulent information, and extensively use mobile payment channels to carry out gambling scams. We conducted a systematic empirical study based on ground-truth data from gambling fraud cases, involving 1,461 fraud case records and 1,487 gambling scam apps from 2021. By carrying out qualitative and quantitative analysis on this ground-truth dataset, we effectively explained the operating channels and fraud chains of gambling scams, which helps to better understand the current gambling scam ecosystem and propose potential countermeasures.
Bio:His research focuses on detection of internet underground industry, cybercrime, privacy protection in mobile applications, and blockchain security. His work has been successfully applied in law enforcement agencies and industry, and he has received honors such as ACM CCS 2018 Highlight Award. Personal webpage: https://ghong.site/